Unlocking AI Readiness: GKN Aerospace's Approach to Effective Governance
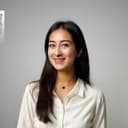
By Salima Mangalji
Published on April 23, 2024
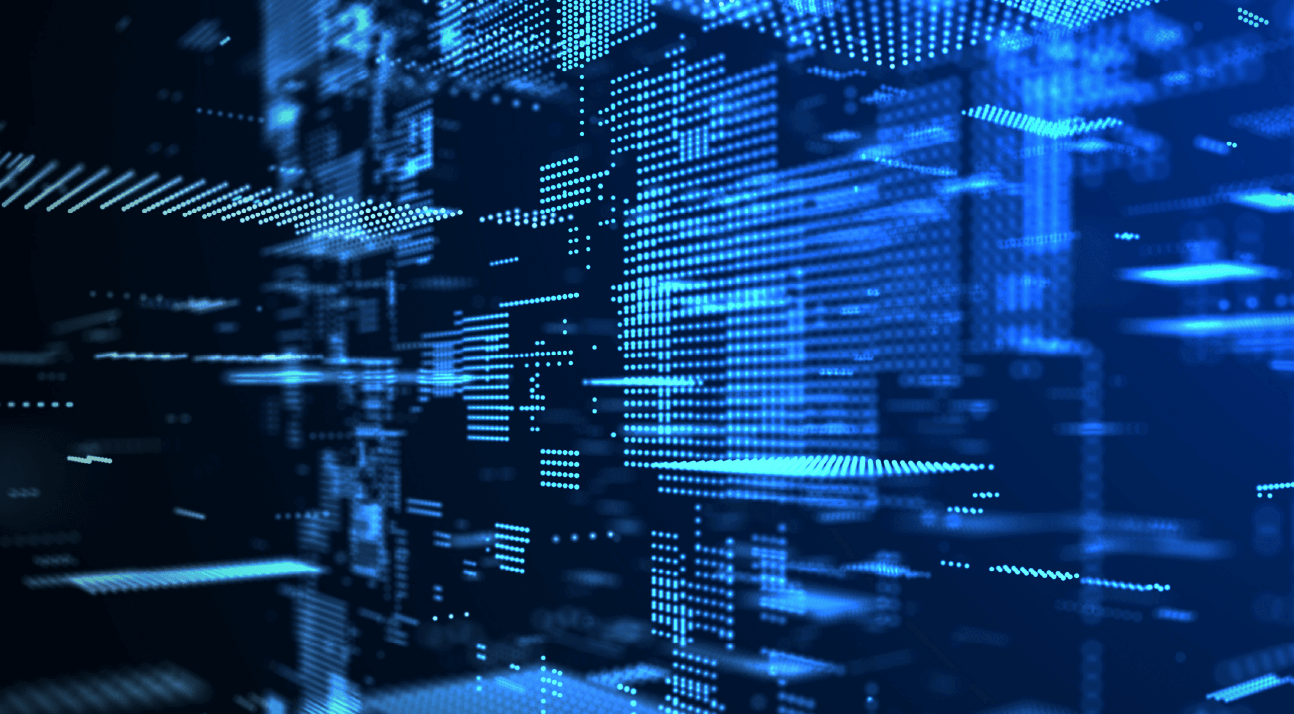
The rise of AI offers enormous untold benefits. Yet, effective governance is critical as organizations scale their AI and data initiatives, especially in highly regulated environments like the aerospace industry. How can data leaders leverage data for maximum impact from AI? We sat down with Alexander Grima, Data & Analytics Architect at GKN Aerospace to learn the answer.
Grima, who has a rich background in rocket science and orbital mechanics, has spearheaded data transformation at GKN. He has worked in the data team at GKN Aerospace for the past seven years, driving transformation in building their own IoT platform, in-house AI and data models, and architectures to serve the organization. Our conversation covered topics such as the global nature of AI governance across various geographies and the challenges of implementing AI due to data limitations in aerospace, such as small data sets, regulations, and compliance considerations. The true differentiator for organizations is not the volume of data they can access but how effectively they can govern that data to maximize its value, deriving meaning and business results from its analysis. Alation stands at the forefront of this paradigm, transforming data into a pivotal asset for innovation in AI/ML initiatives, decision-making, and strategic advantage.
Here are our top 3 takeaways from our conversation:
1. “We’ve got to be very selective with the models that we use. We can’t simply throw data at a black box and hope that it finds correlation.”
Simply feeding large amounts of data into a complex "black box" model and expecting it to produce meaningful results is not a reliable approach. There is a need to understand the underlying mechanisms and assumptions of the models being used, as well as to consider the quality and relevance of the data being inputted. Because the aerospace sector is marked by rigorous regulatory demands, understanding the traceability and mechanics behind AI models is crucial. As a global leader operating in this highly sensitive field, the necessity for stringent data safety and compliance measures, including export control and national security, cannot be understated. Grima’s team relies on people, technology, processes, and governance to enable compliant data and model usage.
Unlike larger-scale manufacturing sectors like plastics or automotive, aerospace production volumes are notably smaller, resulting in limited datasets available for use. This unique challenge requires Grima’s team to be selective with the type of models used. Traditionally, AI initiatives operate on the assumption that there is a large pool of data to pull from (for example, ChatGPT was trained on 45 TB of text data, or 6.5 million pages of text documents). Despite these data constraints, the GKN team is still able to effectively leverage AI, statistics, and machine learning initiatives. This approach not only highlights the team’s adaptability but also reinforces the importance of a robust governance framework to navigate the complexities of the aerospace sector.
2. “How do we enable the experts in the business, as opposed to just bringing out solutions?”
In his seven years leading the data team, Grima has been focused on driving transformation and accelerating their development cycle. Around 18 months ago, Grima spent a lot of time with data owners and stewards trying to understand the data. He realized the data owners possessed ample tribal knowledge and spent much of their time educating people about the data. When processes were to be repeated, people struggled to find the metadata and contextual information they needed. That is when the team brought in Alation to accelerate their development cycle and ensure they were not repeating questions to experts. As a place to store valuable metadata, Alation has helped people answer questions like:
“How is this data being used?”
“What data exists?”
“What’s this table being used for at the moment?”
“What do these columns mean?”
One of the biggest wins for Grima’s team is the ability to build cross-functional, collaborative teams that can work together and understand each other's needs so that they can deliver more quickly. At GKN, there is a data governance manager who heads a data council. The council consists of data owners and a selection of stewards who utilize the data intelligence platform. With GKN’s data governance manager and council, the team fosters an inclusive and collaborative environment to allow data owners and stewards to share experiences and learnings and discuss their utilization of Alation. Alation’s data intelligence platform has also allowed them to document key contextual information, which means that when a user comes back to the data weeks later, they will be able to understand its history and usage more completely. Having a single source of truth to find the meaning of things is crucial. Alation acts as the brain of institutional memory, empowering teams to deliver better solutions faster.
3. “As we start to build more complex models, pipelines, and more complexity into the data lifecycle, how do we retain trust?... How do we trust the models that we use?”
Trusted data is a critical foundation for trusted models and pipelines. But how do you facilitate trust in data? At GKN, data users can explore the data powering an AI model in Alation. They no longer need to rely on data experts or teams to explain the data to them. For example, through Alation, users can see if a specific dataset has a policy attached to it. If that dataset is being consumed by an AI model that does not have a policy attached to it, data owners are alerted.
What's more, data leaders can manage governance through policies, descriptions, and lineage in Alation. They can actively monitor data and models in real time. In this way, a well-governed data intelligence environment creates a solid foundation for trust and transparency. Data leaders need this so they know what is flowing into their models and can better manage the output.
With the advancement of AI moving as quickly as it is now, AI readiness is just as crucial as the technology itself. At the core of successful AI initiatives is a mature data culture. By making data AI-ready—easily discoverable, well-understood, properly governed, and trusted—organizations lay the foundation for these initiatives to thrive. AI models require specific high-quality datasets to learn effectively and make accurate predictions. When trusted data is easily discoverable, teams can move quickly to AI model development and experimentation.
A culture that prioritizes data literacy and leadership ensures that AI initiatives are aligned with strategic business goals and leveraged for maximum impact. A strong data culture not only maximizes the value of existing data assets but also empowers organizations to harness the transformative potential of AI, driving innovation and sustaining competitive advantage. The aerospace industry presents unique challenges, particularly when implementing AI initiatives that demand a strong foundation of well-governed and contextual data. Achieving AI readiness in this sector hinges on the ability to accurately locate the right data sets and ensure that the data fueling models are both trustworthy and compliant with stringent regulations.
Alation’s Data Intelligence Platform facilitates these AI initiatives by offering contextual data intelligence, which forms the basis for a mature data culture. The platform enhances accessibility to trusted data, ensures robust AI governance, and fosters collaborative intelligence. For a deeper exploration of AI Governance within the aerospace industry with Alexander Grima, watch the full webinar.
- 1. “We’ve got to be very selective with the models that we use. We can’t simply throw data at a black box and hope that it finds correlation.”
- 2. “How do we enable the experts in the business, as opposed to just bringing out solutions?”
- 3. “As we start to build more complex models, pipelines, and more complexity into the data lifecycle, how do we retain trust?... How do we trust the models that we use?”